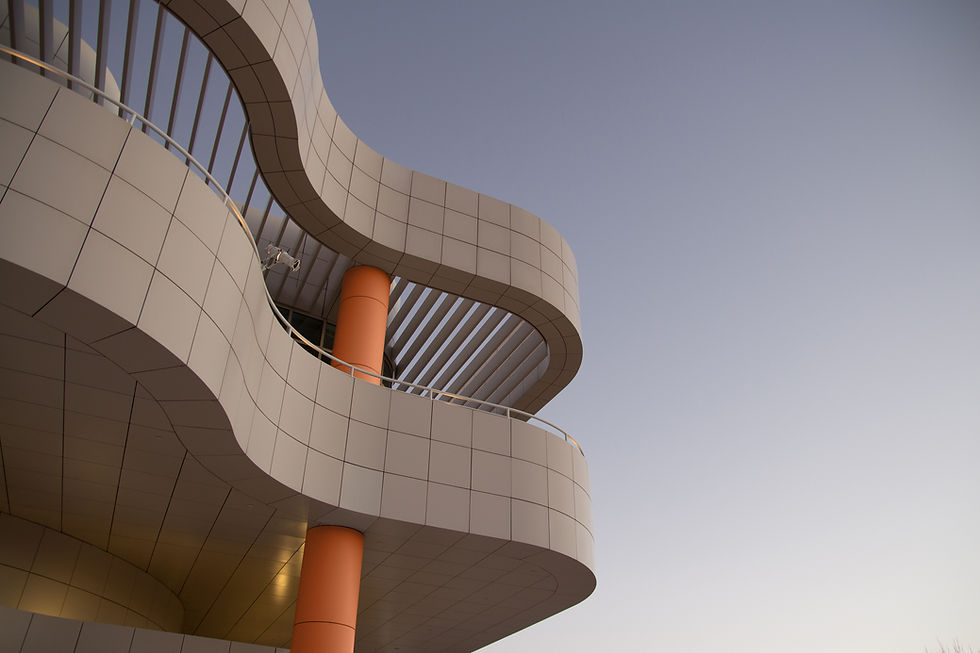
In the realm of philosophy, the question of causation is one of the most fundamental and enduring. Understanding what it means for one event to cause another has profound implications not just for philosophy but for science, law, and everyday reasoning. Among the various approaches to causation, counterfactual theories hold a particularly intriguing position. These theories explore how causation can be understood through the lens of "what if" scenarios—examining what would happen if certain conditions were different.
Understanding Counterfactuals
At its core, a counterfactual statement considers a hypothetical situation that is contrary to the actual events. For instance, consider the statement: "If the match had been struck, it would have lit." Even if the match was not struck and therefore did not light, the counterfactual allows us to reason about what would have happened under different circumstances. This type of reasoning is ubiquitous in our daily lives and is critical to decision-making, moral judgments, and even scientific discovery.
Counterfactual theories of causation build on this type of reasoning. They propose that one event can be said to cause another if, had the first event not occurred, the second event would not have occurred either. This approach contrasts with regularity theories of causation, which focus on the consistent association between types of events, and with mechanistic theories, which emphasize the physical or logical processes linking causes and effects.
In mathematical terms, this can be expressed as:
Causality: A → B if B(not A) ≠ B(A)
The Development of Counterfactual Theories
The roots of counterfactual theories can be traced back to David Hume's analysis of causation in the 18th century. Hume argued that our concept of causation is based on the observation of constant conjunction—when we repeatedly observe that one type of event follows another, we infer a causal connection. However, Hume also acknowledged the role of the mind in making this inference, hinting at a more complex understanding of causation that goes beyond mere observation.
The counterfactual approach was formally developed in the 20th century, particularly through the work of philosophers like David Lewis. Lewis's seminal 1973 paper, "Causation," introduced a rigorous framework for analyzing causation in terms of counterfactuals. According to Lewis, an event A causes an event B if and only if, in the closest possible world where A does not occur, B does not occur either. This formulation brought a new level of precision to the analysis of causation, allowing philosophers to address complex cases that were problematic for other theories.
The Role of Possible Worlds
One of the key innovations in counterfactual theories is the use of possible worlds—a concept borrowed from modal logic. Possible worlds are complete and consistent ways the world might have been. When we evaluate a counterfactual statement, we imagine a possible world that is as similar as possible to the actual world, except for the difference introduced by the counterfactual condition.
For example, when considering the counterfactual "If the match had been struck, it would have lit," we imagine a possible world in which everything is the same as the actual world, except that the match is struck. If in this possible world the match lights, then the counterfactual is true, and we have grounds to say that striking the match would have caused it to light.
This reliance on possible worlds allows counterfactual theories to handle a wide range of causal scenarios, including those involving complex interactions, overdetermination, and preemption. However, it also introduces challenges, particularly in defining the criteria for selecting the "closest" possible world.
Formalizing Counterfactual Causation
To understand the counterfactual relationship mathematically, we often use the notation from structural equation modeling (SEM). In SEM, a causal relationship between variables can be represented by a set of structural equations. For example:
B = f(A, U)
In the context of counterfactual reasoning, we can express the counterfactual outcome B(~A) as:
B(~A) = f(~A, U)
If the difference between B(A) and B(not A) is significant, then A is considered a cause of B.
This relationship can be visualized using causal diagrams, often referred to as Directed Acyclic Graphs (DAGs). Here's a simple DAG illustrating the causal relationship:
A → B
Challenges and Criticisms
While counterfactual theories offer a powerful framework for understanding causation, they are not without their challenges. One of the main criticisms is the problem of indeterminacy in selecting the closest possible world. Different criteria for similarity can lead to different conclusions about causation, raising questions about the objectivity of counterfactual analyses.
For instance, consider a scenario where a tree falls in a forest and causes a fire. If we imagine a possible world where the tree does not fall, we might still have a fire caused by a lightning strike. Does this mean the tree falling was not the cause of the fire? The counterfactual theory would require us to carefully consider which aspects of the actual world are most relevant to the causal relationship, but this can be a highly subjective judgment.
Another challenge is the problem of causal loops, where an event causes itself indirectly. In such cases, counterfactual reasoning can become circular, making it difficult to establish clear causal relationships. For example, if A causes B, and B causes A, counterfactual analysis might struggle to determine which event is the cause and which is the effect.
Moreover, some philosophers argue that counterfactual theories are too reliant on hypothetical scenarios and fail to capture the concrete, physical mechanisms that underlie causal relationships. Critics from the mechanistic perspective, for example, argue that understanding causation requires more than just imagining different possible worlds; it requires an understanding of the actual processes that connect events in the real world.
Statistical Approaches to Counterfactuals
In addition to philosophical discourse, counterfactual reasoning is foundational in statistical approaches to causal inference. The Rubin Causal Model (RCM), also known as the potential outcomes framework, provides a rigorous statistical approach to counterfactual reasoning. In RCM, causality is defined in terms of potential outcomes:
Yᵢ(A) and Yᵢ(~A).
This framework underlies many modern approaches to causal inference in fields such as epidemiology, economics, and social sciences, where randomized experiments or natural experiments are used to estimate these potential outcomes.
Applications and Implications
Despite these challenges, counterfactual theories of causation have found important applications in various fields. In law, for example, counterfactual reasoning is often used to assess liability. Courts frequently ask whether harm would have occurred "but for" the defendant's actions—a question that closely mirrors the counterfactual analysis of causation.
In science, counterfactual reasoning is central to experimental design and causal inference. Randomized controlled trials, for instance, are designed to approximate counterfactual conditions by comparing outcomes between treated and untreated groups. Similarly, in epidemiology and economics, counterfactual models are used to estimate the causal effects of interventions, policies, or treatments.
In everyday life, counterfactual reasoning helps us make sense of our experiences, learn from our mistakes, and plan for the future. We constantly evaluate the consequences of our actions by considering what might have happened if we had acted differently. This kind of reasoning is crucial for moral and practical decision-making, allowing us to navigate a complex and uncertain world.
The Future of Counterfactual Theories
Looking ahead, counterfactual theories of causation are likely to continue evolving as philosophers address their limitations and explore new applications. One promising direction is the integration of counterfactual reasoning with probabilistic and statistical approaches to causation. By combining the strengths of different causal theories, it may be possible to develop a more comprehensive and robust framework for understanding causation in both philosophy and science.
Another area of interest is the role of counterfactuals in artificial intelligence and machine learning. As AI systems become more advanced, they increasingly rely on counterfactual reasoning to make decisions, explain their actions, and optimize their performance. Understanding the philosophical foundations of counterfactuals could provide valuable insights for the development of more transparent and trustworthy AI systems.
In conclusion, counterfactual theories of causation offer a compelling and versatile approach to understanding one of the most fundamental aspects of our world. While they face significant challenges and criticisms, their ability to capture the nuances of causation in diverse contexts makes them an indispensable tool for philosophers, scientists, and anyone seeking to understand how the world works. As we continue to explore the complexities of causation, counterfactual reasoning will undoubtedly remain at the forefront of philosophical inquiry, offering new perspectives and insights into the nature of cause and effect.